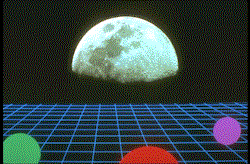 |
WSC 2002 Final Abstracts |
Monday 10:30:00 AM 12:00:00 PM
Simulation in Financial Engineering
Chair: John Charnes (University of Kansas)
Simulation in Financial Engineering
Jeremy
Staum (Cornell University)
Abstract:
This paper presents an overview of the use of
simulation algorithms in the field of financial engineering, assuming on the
part of the reader no familiarity with finance and a modest familiarity with
simulation methodology, but not its specialist research literature. The focus
is on the challenges specific to financial simulations and the approaches that
researchers have developed to handle them, although the paper does not
constitute a comprehensive survey of the research literature. It offers to
simulation researchers, professionals, and students an introduction to an
application of increasing significance both within the simulation research
community and among financial engineering practitioners.
Monday 1:30:00 PM 3:00:00 PM
Derivatives and Credit Risk
Chair: Paul Na (Bayerische Landesbank New York
Branch)
Importance Sampling for Multimodal Functions and
Application to Pricing Exotic Options
Athanassios N. Avramidis
(University of Montreal)
Abstract:
We consider importance sampling (IS) to increase the
efficiency of Monte Carlo integration, especially for pricing exotic options
where the random input is multivariate Normal. When the importance function
(the product of integrand and original density) is multimodal, determining a
good IS density is a difficult task. We propose an Automated Importance
Sampling Density Estimation Procedure (AISDE). AISDE selects an IS density as
a mixture of multivariate Normal densities with modes at certain local maxima
of the importance function. When the simulation input is multivariate Normal,
we use principal component analysis to obtain a reduced-dimension, approximate
importance function, which allows efficient identification of a good IS
density via AISDE in original problem dimensions over 100. We present Monte
Carlo experimental results on randomly generated option-pricing problems
(including path-dependent options), demonstrating large and consistent
efficiency improvement.
Enhanced Quasi-Monte Carlo Methods with Dimension
Reduction
Junichi Imai (Iwate Prefectural University) and Ken Seng
Tan (University of Waterloo)
Abstract:
In recent years, the quasi-Monte Carlo approach for
pricing high-dimensional derivative securities has been used widely relative
to other competitive approaches such as the Monte Carlo methods. Such success
can be, in part, attributed to the notion of effective dimension of the
finance problems. In this paper, we provide additional insight on the
connection between the effective dimension and the quasi-Monte Carlo method.
We also propose a dimension reduction technique which further enhances the
quasi-Monte Carlo method for derivative pricing. The efficiency of the
proposed method is illustrated by applying it to high-dimensional multi-factor
path-dependent derivative securities.
Credit Risk Modeling for Catastrophic
Events
Tarja Joro (University of Alberta School of Business) and
Paul Na (Bayerische Landesbank New York Branch)
Abstract:
Estimating default probabilities of companies is one of
the fundamental tasks in credit risk models and lending decision-making. One
area of particular interest is how the companies’ asset value behaves in the
presence of unforeseen external shocks or catastrophes. On one hand, we want
the default probabilities to address the likelihood of catastrophes correctly,
and on the other hand, we want to be able to perform what-if analysis to
investigate the possible consequences of catastrophes. This study proposes a
framework to perform such what-if analysis in the jump diffusion framework.
Monday 3:30:00 PM 5:00:00 PM
Asset Price Modeling
Chair: Tarja
Joro (University of Alberta)
A Spot Pricing Framework to Enable Pricing and Risk
Management of Inter-Domain Assured Bandwidth Services
Mehdi
Aboulfadl, Aparna Gupta, Ritesh Pradhan, and Shivkumar Kalyanaraman
(Rensselaer Polytechnic Institute)
Abstract:
In the current bandwidth market, Internet Service
Providers (ISPs) provide guaranteed Internet bandwidth within their domains.
However, they are incapable of providing such assurances for data crossing
their domain boundaries. In this paper, we present a spot pricing scheme for
Internet bandwidth contracts within an ISP domain. These models when
implemented at access or exchange points of different ISP domains would
provide assured bandwidth for inter-domain traffic. Each contract will
constitute a Quality of Service agreement between a customer and a provider
within an ISP domain. By appropriately bundling derivative contracts defined
on the intra-domain service contracts, a provider will not only be able to
give inter-domain Quality of Service assurance, but will be able to add new
services and manage its portfolio of services.
Modeling Growth Stocks (Part II)
Samuel Kou
(Harvard University) and Steve Kou (Columbia University)
Abstract:
Continuing the previous work on growth stocks, we
propose a diffusion model for growth stocks. Since growth stocks tend to have
low or even negative earnings and high volatility, it is a great challenge to
derive a meaningful mathematical model within the traditional valuation
framework. The diffusion model not only has economic interpretations for its
parameters, but also leads to some interesting economic insight - the model
postulates mean reversion (with a high mean reverting level) for growth
stocks, which could be useful in understanding the recent boom and burst of
the "internet bubble". Simulation and an empirical evaluation of the model
based on the size distribution are also presented. The simulation and
numerical results are quite encouraging.
Decision Aids for Scheduling and Hedging (DASH) in
Deregulated Electricity Markets: A Stochastic Programming Approach to Power
Portfolio Optimization
Suvrajeet Sen, Lihua Yu, and Talat Genc
(University of Arizona)
Abstract:
The DASH model for Power Portfolio Optimization
provides a tool which helps decision-makers coordinate production decisions
with opportunities in the wholesale power market. The methodology is based on
a stochastic programming model which selects portfolio positions that perform
well on a variety of scenarios generated through statistical modeling and
optimization. When compared with a commonly used fixed-mix policy, our
experiments demonstrate that the DASH model provides significant advantages
over several fixed-mix policies.
Tuesday 8:30:00 AM 10:00:00 AM
Crystal Ball Software Tutorial
Chair: James Kelly (OptTek Systems, Inc.)
Crystal Ball Professional Introductory
Tutorial
Lawrence I. Goldman (Decisioneering, Inc.)
Abstract:
Crystal Ball 2000 Professional Edition is a suite of
easy-to-use Microsoft Excel add-in software that helps you analyze the risks
and uncertainties associated with your spreadsheet models. The suite includes
analysis tools for Monte Carlo simulation (Crystal Ball), time-series
forecasting (CB Predictor), and optimization (OptQuest) as well as developer
kits for building custom interfaces and processes. Spreadsheets alone are
inadequate for assessing the probability of an event because they lack the
ability to generate and analyze alternative scenarios in a sophisticated way.
Spreadsheet add-ins such as Crystal Ball can provide this functionality and
help modelers gain new insights into the mechanisms that drive their models
and affect positive outcomes. This tutorial uses the example of an emerging
media product to discuss how the analytical tools of Monte Carlo simulation
and time-series forecasting can provide a greater understanding and
quantification of the risks inherent in a spreadsheet-based business decision.
Tuesday 10:30:00 AM 12:00:00 PM
OptQuest Software Tutorial
Chair: Jay April (OptTek Systems, Inc.)
Portfolio Optimization for Capital Investment
Projects
Jay April, Fred Glover, and James Kelly (OptTek Systems,
Inc.)
Abstract:
The new portfolio optimization engine, OptFolio™,
simultaneously addresses financial return goals, catastrophic loss avoidance,
and performance probability. The innovations embedded in OptFolio enable users
to confidently design effective plans for achieving financial goals, employing
accurate analysis based on real data. Traditional analysis and prediction
methods are based on mean variance analysis - an approach known to be faulty.
OptFolio takes a much more sophisticated and strategic direction.
State-of-the-art technology integrates optimization and simulation techniques
and a new surface methodology based on linear programming into a global system
that guides a series of evaluations to reveal truly optimal investment
scenarios. OptFolio is currently being used to optimize project portfolio
performance in oil and gas applications and in capital allocation and
budgeting for investments in technology.
Tuesday 1:30:00 PM 3:00:00 PM
Problems in Financial Engineering
Chair: Stewart Mayhew (University of Georgia)
Optimal Active Management Fees
Jakša
Cvitanic (University of Southern California) and Lionel Martellini and
Fernando Zapatero (USC)
Abstract:
We consider the problem of a mutual fund manager that
maximizes the present value of expected fees and has to decide the level of
fee to impose on the fund. The fee will be paid by a risk averse investor that
maximizes expected utility over final wealth. This investor can invest either
in an indexed fund or in a managed fund. The manager has superior ability and,
as a result of it, the fund offers a higher expected return. However, the
investor has incomplete information about the ability of the fund manager. The
investor has priors about this ability that are upgraded according to the
performance of the fund. At some optimal level, the investor decides to switch
from the market portfolio to the mutual fund. Our problem does not have a
closed form solution, but we can compute optimal fees, using simulation.
Convergence of the Stochastic Mesh Estimator for
Pricing American Options
Athanassios N. Avramidis (University of
Montreal) and Heinrich Matzinger (Universtity of Bielefeld )
Abstract:
Broadie and Glasserman proposed a simulation-based
method they named stochastic mesh for pricing high-dimensional American
options. Based on simulated states of the assets underlying the option at each
exercise opportunity, the method produces an estimator of the option value at
each sampled state. Under the mild assumption of the finiteness of certain
moments, we derive an asymptotic upper bound on the probability of error of
the mesh estimator, where both the error size and the probability bound vanish
as the sample size increases. We include the empirical performance for the
test problems used by Broadie and Glasserman in a recent unpublished
manuscript. We find that the mesh estimator has large bias that decays very
slowly with the sample size, suggesting that in applications it will most
likely be necessary to employ bias and/or variance reduction techniques.
Security Price Dynamics and Simulation in Financial
Engineering
Stewart Mayhew (University of Georgia)
Abstract:
Applications in financial engineering have relied
heavily on Brownian Motion as a workhorse model for pricing derivative
securities and implementing risk management programs. When more than one state
variable is required, the standard approach is to use a multivariate Brownian
Motion with constant correlations. This article briefly summarizes several
important reasons why this approach is not adequate (and in some cases, can
lead to disaster). Examples include fat tails, volatility clustering, large
discrete jumps, parameter instability, and asymmetric correlations. Including
such features makes analytic modeling less tractable, and potentially makes
simulation a more attractive alternative.
Tuesday 3:30:00 PM 5:00:00 PM
Energy Production and Trading
Chair: Athanassios N. Avramidis (University of
Montreal)
Using Computer Simulation to Mitigate Risk in
Electricity Generation/Consumption Collaboration Policies
Thomas F.
Brady (Purdue University North Central)
Abstract:
The electric utility industry has undergone fundamental
change in the last decade. Foremost of these changes have been numerous
deregulation attempts. Producers and large consumers have built business
models based upon large volume transactions, which lead to smooth production
and volume discounting. The risks associated with using these traditional
business models in deregulated markets are many. This paper describes the
development of a computer simulation environment that models a novel
collaborative strategy proposed by a local electricity utility to mitigate
highly varying load situations demanded by the largest steel-producing region
in the United States. Through the use of this model, collaborative strategies
for effective electricity generation and usage are developed and analyzed.
Batting Average: A Composite Measure of Risk for
Assessing Product Differentiation in a Simulation Model
Daniel M.
Hamblin (Dan Hamblin & Associates, Inc.) and Brian T. Ratchford
(University of Maryland)
Abstract:
The paper simulates how market power affects
electricity retailing to households. A pseudo-random number seeding algorithm
creates representative product differentiation in repeated drawings, for an
incumbent and seven challengers. A ninth player competitor decides how to
distinguish her product. The simulation creates an efficient starting market,
adjusted for competitor dominance; and, over a 12-month horizon, uses topology
to develop unexploited profit opportunities for all competitors. A best
solution criterion punishes nonconformists. Results of repeated drawings
varying opposition to the player's constant product differentiation feed a
batting average risk assessment. Decision rules reward hits based on profit
and year's end market share. The market simulation tool supports conjectural
assessment of social policy - household direct access to wholesale power,
incentive for product differentiation versus that for mergers and
acquisitions, and allocation of deregulation benefits to shareholders versus
ratepayers.
Discrete Event Simulation for the Risk of Development
of an Oil Field
Carlos Magno C. Jacinto (PETROBRAS SA)
Abstract:
The present work focuses on the development of a
simulation method which provides an engineering tool for managing the risks
associated with the development of an oil field. The developed method consists
of performing discrete simulation based on data from field operations. The
paper reports and discusses the simulation results of a real field development
which lead to the following highlights: (1) the operations that present the
highest level of risk are emphasized and studied for defining their impact on
costs, (2) the consequences of some deviations from the pre-planned schedule
are evaluated in terms of increasing the total amount of the investment, and
(3) the possible gains and risks associated with the use of some emerging
technologies are analyzed. This study shows that this methodology is an useful
tool for providing relevant information to contracts negotiation with
suppliers and drilling contractors.
Wednesday 8:30:00 AM 10:00:00 AM
Financial Derivatives and Real
Options
Chair: Michael Fu (University of
Maryland)
Hedging Beyond Duration and Convexity
Jian
Chen (Fannie Mae) and Michael C. Fu (University of Maryland)
Abstract:
Hedging of fixed income securities remains one of the
most challenging problems faced by financial institutions. The predominantly
used measures of duration and convexity do not completely capture the interest
rate risks borne by the holder of these securities. Using historical data for
the entire yield curve, we perform a principal components analysis and find
that the first four factors capture over 99.99% of the yield curve variation.
Incorporating these factors into the pricing of arbitrary fixed income
securities via Monte Carlo simulation, we derive perturbation analysis (PA)
estimators for the price sensitivities with respect to the factors.
Computational results for mortgage-backed securities (MBS) indicate that using
these sensitivity measures in hedging provides far more protection against
interest risk exposure than the conventional measures of duration and
convexity.
Effect of Implementation Time on Real Options
Valuation
Harriet Black Nembhard, Leyuan Shi, and Mehmet Aktan
(University of Wisconsin-Madison)
Abstract:
Exercising real options often requires an
implementation time, whereas financial options can be exercised instantly.
Neglecting the implementation time needed to exercise a real option causes
overvaluing that option. We develop lattice and Monte Carlo simulation
techniques to value real option problems, where exercising the option requires
an implementation time. We present the application of the proposed techniques
on a global supply chain network problem with exchange rate uncertainty and
value the flexibility to switch between manufacturing options for a firm that
has operations in different countries.
An Empirical Evaluation of Sampling Methods in Risk
Analysis Simulation: Quasi-Monte Carlo, Descriptive Sampling, and Latin
Hypercube Sampling
Eduardo Saliby (COPPEAD-UFRJ) and Flavio Pacheco
(Banco Boreal de Investimentos S/A)
Abstract:
This paper compares the performance, in terms of
convergence rates and precision of the estimates, for six Monte Carlo
Simulation sampling methods: Quasi-Monte Carlo using Halton, Sobol, and Faure
numeric sequences; Descriptive Sampling, based on the use of deterministic
sets and Latin Hypercube Sampling, based on stratified numerical sets. Those
methods are compared to the classical Monte Carlo. The comparison was made for
two basic risky applications: the first one evaluates the risk in a decision
making process when launching a new product; the second evaluates the risk of
accomplishing an expected rate of return in a correlated stock portfolio.
Descriptive sampling and Latin Hypercube sampling have shown the best
aggregate results.
![]()