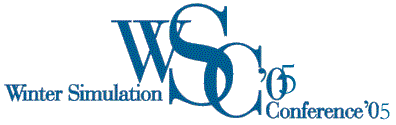 |
WSC 2005 Final Abstracts |
Monday 10:30:00 AM 12:00:00 PM
Credit Risk Analysis
Chair:
Michael Fu (Maryland)
The Delivery Option in Mortgage Backed Security
Valuation Simulations
Scott Gregory Chastain and Jian Chen (Fannie
Mae)
Abstract:
A delivery option exists in mortgage-backed security
market, which has not been considered in existing mortgage pricing simulation
literature. We explain the delivery option, the "To Be Announced" trade. We
discuss how the presence of the delivery option effects the use of the
standard pricing simulation technique. This technique uses a risk neutral
interest rate simulation with a prepayment option model to recover a price
which is an expectation over the possible rate outcomes. The simulation
technique uses Monte Carlo integration with a suitable selected pseudo or
quasi-random sequence. To recover market prices a spread term called the
"Option Adjusted Spread" is required. We see that multiple simulations are
required to explore the full structure of the delivery option but suggest how
to use one simulation to approximate pricing even when the delivery option is
present.
Simulation Analysis of Correlation and Credit
Migration Models for Credit Portfolios
William J. Morokoff (Moody’s
KMV)
Abstract:
The market for derivatives such as first-to-default
baskets and CDO tranches on portfolios of corporate credit exposures (bonds,
loans, default swaps, etc.) has grown rapidly in recent years. Various models
for capturing portfolio correlation effects have been introduced, with Default
Time models becoming the most widely used. While attractive for their relative
simplicity and ability, in some cases, to allow fast computation of hedge
ratios, there is increasing concern around the limitations and implications of
these models. This paper uses simulation to study the effects of credit
migration and correlation assumptions underlying the models for valuation of
derivatives on credit portfolios.
A Loss Default Simulation Model of the Federal Bank
Deposit Insurance Funds
Rosalind L. Bennett, Daniel A and Nuxoll
(FDIC), Robert A. Jarrow (Cornell University) and Michael C. Fu and Huiju
Zhang (University of Maryland)
Abstract:
This paper discusses a simulation model that is used in
a martingale valuation approach to measure and value the risk of the FDIC
deposit insurance funds. The FDIC insurance funds capitalize a portfolio of
insurance policies, each issued to depositors of an individual commercial
bank. To evaluate this portfolio, our methodology evaluates the insurance
policies for depositors at each individual bank and aggregates to obtain the
risk of the entire portfolio. To adequately model the risks associated with
credit, interest rate, deposit growth, and loss rate, a multi-dimensional
system is formulated. The risk measurement and valuation results are based on
Monte Carlo simulation of the system risks.
Monday 1:30:00 PM 3:00:00 PM
Simulation Methodology for Credit Risk
Models
Chair: Perwez Shahabuddin (Columbia
University)
Simulation of Risk and Return Profiles for
Portfolios of CDO Tranches
William J. Morokoff (Moody's KMV)
Abstract:
Investments in Collateralized Debt Obligations (CDOs)
often offer attractive yields relative to other similar debt instruments
(corporate bonds, etc.). However, the risk profiles of CDO investments, and in
particular portfolios of these investments, can be substantially different
from straight credit portfolios due to complex correlation dependence across
CDOs. Simulation is generally required to capture the intricate interaction of
default and correlation risk that determines the risk and return profile of a
portfolio of CDO investments. This paper considers some of the issues that
must be addressed in determining the risk profiles with simulation and
presents results on a simple example.
Expected Shortfall in Credit Portfolios with
Extremal Dependence
Achal Bassamboo (Kellog School of Management),
Sandeep Juneja (Tata Institute of Fundamental Research) and Assaf Zeevi
(Columbia University)
Abstract:
We consider the risk of a portfolio comprised of loans,
bonds, and financial instruments that are subject to possible default. We are
interested in efficiently estimating expected excess loss conditioned on the
event that the portfolio incurs large losses over a fixed time horizon; this
risk measure is often referred to as expected shortfall. We consider a
heterogeneous mix of obligors and assume a portfolio dependence structure that
supports extremal dependence among obligors and does not hinge solely on
correlation. We first derive sharp asymptotics that illustrate the
implications of extremal dependence among obligors in the risk of the
portfolio. Using this as a stepping stone, we develop a multi-stage importance
sampling algorithm that is shown to have bounded relative error in estimating
expected shortfall.
Fast Simulation of Multifactor Portfolio
Credit Risk in the t-Copula Model
Wanmo Kang (Moody's KMV) and
Perwez Shahabuddin (Columbia University)
Abstract:
We present an importance sampling procedure for the
estimation of multifactor portfolio credit risk for the t-copula model, i.e,
the case where the risk factors have the multivariate student t distribution.
We use a version of the multivariate student t that can be expressed as a
ratio of a multivariate normal and a scaled chi-square random variable. The
procedure consists of two steps. First, using the large deviations result for
the Gaussian model in Glasserman, Kang, and Shahabuddin (2005a), we devise and
apply a change of measure to the chi-square random variable. Then, conditional
on the chi-square random variable, we apply the importance sampling procedure
developed for the Gaussian-copula model in Glasserman, Kang, Shahabuddin
(2005b). We support our importance sampling procedure by numerical examples.
Monday 3:30:00 PM 5:00:00 PM
Risk Analysis Methodology
Chair:
Sam Ehrlichman (Cornell University)
Out-of-the-money Monte Carlo Simulation Option
Pricing: The Joint Use Of Importance Sampling And Descritptive
Sampling
Eduardo Saliby, Jaqueline T.M. Marins, and Josete F. dos
Santos (Coppead/UFRJ)
Abstract:
As in any Monte Carlo application, simulation option
valuation produces imprecise estimates. In such an application, Descriptive
Sampling (DS) has proven to be a powerful Variance Reduction Technique.
However, this performance deteriorates as the probability of exercising an
option decreases. In the case of out of the money options, the solution is to
use Importance Sampling (IS). Following this track, the joint use of IS and DS
is deserving of attention. Here, we evaluate and compare the benefits of using
standard IS method with the joint use of IS and DS. We also investigate the
influence of the problem dimensionality in the variance reduction achieved.
Although the combination IS+DS showed gains over the standard IS
implementation, the benefits in the case of out-of-the-money options were
mainly due to the IS effect. On the other hand, the problem dimensionality did
not affect the gains. Possible reasons for such results are discussed.
Function-approximation-based Perfect Control
Variates for Pricing American Options
Sandeep Juneja and Nomesh
Bolia (Tata Institute of Fundamental Research)
Abstract:
Monte Carlo simulation techniques that use function
approximations have been successfully applied to approximately price
multi-dimensional American options. However, for many pricing problems the
time required to get accurate estimates can still be prohibitive, and this
motivates the development of variance reduction techniques. In this paper, we
describe a zero-variance or `perfect' control variate to price American
options. We then discuss how function approximation may be used to approximate
this perfect control variate. Empirically, we observe that on simple one
dimensional examples, this approximately perfect control variate gives orders
of magnitude of variance reduction compared to naive estimation.
A Study of Variance Reduction Techniques for
American Option Pricing
Christiane Lemieux and Jennie La
(University of Calgary)
Abstract:
American option pricing is a challenging problem in
financial mathematics for which several approaches have been proposed in the
last few years. In this paper, we consider the regression-based method of
Longstaff and Schwartz (2001) to price these options, and then investigate the
use of different variance reduction techniques to improve the efficiency of
the Monte Carlo estimators thus obtained. The techniques considered have been
shown to work well for European option pricing. One of them is importance
sampling, in which the approach of Glasserman, Heidelberger and Shahabuddin
(1999) is applied to find an appropriate change of measure. We also consider
control variates and randomized quasi-Monte Carlo methods, and use numerical
experiments on American Asian call options to investigate the performance of
these methods.
Tuesday 8:30:00 AM 10:00:00 AM
Operational Risk Analysis
Chair: Kevin Taaffe (Clemson University)
Risk Assessment of Drilling and Completion
Operations in Petroleum Wells Using a Monte Carlo and a Neural Network
Approach
Dennis Kerr Coelho, Mauro Roisenberg, and Paulo José
Freitas (Federal University of Santa Catarina) and Carlos Magno Jacinto
(Petrobras)
Abstract:
This paper intends to show how two different
methodologies, a Monte Carlo simulation method and a connectionist approach
can be used to estimate the total time assessment in drilling and completion
operations of oil wells in deep waters. The former approach performs a Monte
Carlo simulation based on data from field operations. In the later one,
correlations and regularities in parameters selected from a petroleum company
database were detected using a competitive neural network, and then, a
feedforward neural network was trained to estimate the average, standard
deviation and total time wasted in the accomplishment of the well. At the end,
the results obtained by both models are compared. The analyst could evaluate
the precision of the estimated total-time based on geometric and technological
parameters provided by the neural network tool, with those supplied by the
traditional Monte Carlo method based on data of the drilling and completion
operations
Identifying Demand Sources That Minimize Risk for a
Selective Newsvendor
Kevin M. Taaffe and Deepak Tirumalasetty
(Clemson University)
Abstract:
Consider a firm that offers a product during a single
selling season. The firm has the flexibility of choosing which demand sources
to serve, but these decisions must be made prior to knowing the actual demand
that will materialize in each market. Moreover, we assume the firm operates on
a tight budget and cannot afford to record several successive financial losses
spanning consecutive periods. In this case, it is likely that their objective
is not only to maximize expected profit, but to minimize the variance from
that goal. We provide insights into the tradeoff between expected profit,
expected revenue, and demand uncertainty. Finally, we present a solution
approach, via simulation, to determine the best set of markets to pursue and
the associated order quantity when the firm's objective is to minimize the
probability of receiving a profit below a critical threshold value.
Using Simulation to Analyze R&D Value
Creation
Douglas A. Bodner, William B. Rouse, and Michael J.
Pennock (Georgia Institute of Technology)
Abstract:
As the front-end to product and system lifecycles,
research and development activities serve as engines of value creation. By
nature, though, R&D involves significant uncertainty. As such, it often is
viewed as an investment problem, whereby funds are invested in ventures under
risk, with the hope of achieving future value. This paper investigates the use
of organizational simulation to analyze the R&D investment problem,
focusing on ways to increase value created from R&D. Based on a
process-focused model of R&D systems, initial results indicate that using
a real options framework to valuate R&D outperforms traditional discounted
cash flow (DCF) methods in total value created, but that DCF methods are
preferred for return on R&D investment. To complement the process-focused
R&D system model, a product-focused model of R&D is specified and
integrated with the process-focused model.
![]()