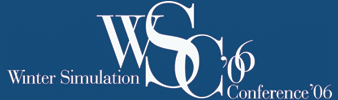 |
WSC 2006 Abstracts |
Tuesday 1:30:00 PM 3:00:00 PM
Pricing American Options
Chair:
Samuel Ehrlichman (Cornell University)
A New Efficient Simulation Strategy for Pricing
Path-Dependent Options
Gang Zhao, Yakun Zhou, and Pirooz Vakili
(Boston University)
Abstract:
The purpose of this paper is twofold. First, it serves
to describe a new strategy, called Structured Database Monte Carlo (SDMC), for
efficient Monte Carlo simulation. Its second aim is to show how this approach
can be used for efficient pricing of path-dependent options via simulation. We
use efficient simulation of a sample of path-dependent options to illustrate
the application of SDMC. Extensions to other path-dependent options are
straightforward.
Applying Model Reference Adaptive Search to
American-Style Option Pricing
Huiju Zhang and Michael Fu
(University of Maryland)
Abstract:
This paper considers the application of stochastic
optimization methods to American-style option pricing. We apply a randomized
optimization algorithm called Model Reference Adaptive Search (MRAS) to
pricing American-style options by parameterizing the early exercise boundary.
Numerical results are provided for pricing American-style call and put options
written on underlying assets following geometric Brownian motion and Merton
jump-diffusion processes. The results from the MRAS algorithm are also
compared with the Cross-Entropy (CE) method.
American Options From Mars
Samuel M.
T. Ehrlichman and Shane G. Henderson (Cornell University)
Abstract:
We develop a class of control variates for the American
option pricing problem that are constructed through the use of MARS --
multivariate adaptive regression splines. The splines approximate the option's
value function at each time step, and the value function approximations are
then used to construct a martingale that serves as the control variate.
Significant variance reduction is possible even in high dimensions. The
primary restriction is that we must be able to compute certain one-step
conditional expectations.
Tuesday 3:30:00 PM 5:00:00 PM
Risk Analysis
Chair: Jeremy
Staum (Northwestern University)
Using Copulas in Risk Analysis
Dalton F
Andrade, Pedro Barbetta, Paulo José Freitas, and Ney A. M. Zunino (Federal
University of Santa Catarina - UFSC) and Carlos Magno Jacinto (Petrobras)
Abstract:
Practically every well installation process nowadays
relies on some sort of risk assessment study, given the high costs involved.
Those studies focus mostly on estimating the total time required by the well
drilling and completion operations, as a way to predict the final costs. Among
the different techniques employed, the Monte Carlo Simulation currently stands
out as the preferred method. One relevant aspect which is frequently left out
from simulation models is the dependence relationship among the processes
under consideration. That omission can have a serious impact on the results of
risk assessment and, consequently, on the conclusions drawn from them. In
general, practitioners do not incorporate the dependence information because
that is not always an easy task. This paper intends to show how Copula
functions may be used as a tool to build correlation-aware Monte Carlo
simulation models.
An Adaptive Procedure for Estimating Coherent Risk
Measures Based on Generalized Scenarios
Vadim Lesnevski, Barry L.
Nelson, and Jeremy Staum (Northwestern University)
Abstract:
Coherent risk measures based on generalized scenarios
can be viewed as estimating the maximum expected value from among a collection
of simulated "systems." We present a procedure for generating a fixed-width
confidence interval for this coherent risk measure. The procedure improves
upon previous methods by being reliably efficient for simulation of
generalized scenarios and portfolios with heterogeneous characteristics.
Wednesday 8:30:00 AM 10:00:00 AM
Efficient Simulation for Risk
Management
Chair: Jose Blanchet (Harvard
University)
Efficient Importance Sampling for Reduced Form
Models in Credit Risk
Achal Bassamboo (Kellogg School of
Management) and Sachin Jain (Amaranth Group Inc.)
Abstract:
In this paper we study the problem of estimating
probability of large losses in the framework of doubly stochastic credit risk
models. We derive a logarithmic asymptote for the probability of interest in a
specific asymptotic regime and propose an asymptotically optimal importance
sampling algorithm for efficiently estimating the same. The numerical results
in the last section corroborate our theoretical findings.
Efficient Simulation for Risk Measurement in
Portfolio of CDOs
Michael Gordy (The Federal Reserve Board) and
Sandeep Juneja (Tata Institute of Fundamental Research)
Abstract:
We consider a portfolio containing CDO tranches and
ordinary bonds. Our interest is in large loss probabilities and risk measures
such as value-at-risk. When loss is measured on mark-to-market basis,
estimation via simulation requires a nested procedure: In the outer step one
draws realizations of all risk factors up to the horizon, and in the inner
step one re-prices each instrument in the portfolio at the horizon conditional
on the drawn risk factors. Practitioners perceive the computational burden of
such nested schemes to be unacceptable, and adopt variety of somewhat ad hoc
measures to avoid the inner simulation. In this paper, we question whether
such short cuts are necessary. We show that relatively small number of trials
in the inner step can yield accurate estimates, and analyze how fixed
computational budget may be allocated to inner and outer steps to minimize the
mean square error of the resultant estimator.
Efficient Simulation for Large Deviation
Probabilities of Sums of Heavy-tailed Random Variables
Jose
Blanchet and Jingchen Liu (Harvard University)
Abstract:
We describe an efficient state-dependent importance
sampling algorithm for estimating large deviation probabilities for sums of
iid rv's with finite variance and regularly varying tails. Our algorithm can
be shown to be strongly efficient basically throughout the whole large
deviations region as the number of increments increases. Our techniques
combine results of the theory of large deviations for sums of regularly
varying distributions and the basic ideas can be applied to other rare-event
simulation problems involving both light and heavy-tailed features.
Wednesday 10:30:00 AM 12:00:00 PM
Stochastic Programming in Risk
Analysis
Chair: David Morton (University of Texas at Austin)
The BEST Algorithm for Solving Stochastic Mixed
Integer Programs
Susan Sanchez and Kevin Wood (Naval Postgraduate
School)
Abstract:
We present a new algorithm for solving two-stage
stochastic mixed-integer programs (SMIPs) having discrete first-stage
variables, and continuous or discrete second-stage variables. For a minimizing
SMIP, the BEST algorithm (1) computes an upper Bound on the optimal objective
value (typically a probabilistic bound), and identifies a deterministic
lower-bounding function, (2) uses the bounds to Enumerate a set of first-stage
solutions that contains an optimal solution with pre-specified confidence, (3)
for each first-stage solution, Simulates second-stage operations by repeatedly
sampling random parameters and solving the resulting model instances, and (4)
applies statistical Tests (e.g., "screening procedures") to the simulated
outcomes to identify a near-optimal first-stage solution with pre-specified
confidence. We demonstrate the algorithm's performance on a stochastic
facility-location problem.
Quasi-Monte Carlo Strategies for Stochastic
Optimization
Shane Drew (Northwestern University) and Tito
Homem-de-Mello (Nortwestern University)
Abstract:
In this paper we discuss the issue of solving
stochastic optimization problems using sampling methods. Numerical results
have shown that using variance reduction techniques from statistics can result
in significant improvements over Monte Carlo sampling in terms of the number
of samples needed for convergence of the optimal objective value and optimal
solution to a stochastic optimization problem. Among these techniques are
stratified sampling and Quasi-Monte Carlo sampling. However, for problems in
high dimension, it may be computationally inefficient to calculate Quasi-Monte
Carlo point sets in the full dimension. Rather, we wish to identify which
dimensions are most important to the convergence and implement a Quasi-Monte
Carlo sampling scheme with padding, where the important dimensions are sampled
via Quasi-Monte Carlo sampling and the remaining dimensions with Monte Carlo
sampling. We then incorporate this sampling scheme into an external sampling
algorithm (ES-QMCP) to solve stochastic optimization problems.
Jackknife Estimators for Reducing Bias in Asset
Allocation
Amit Partani and David P Morton (The University of Texas
at Austin) and Ivilina Popova (Seattle University)
Abstract:
We use jackknife-based estimators to reduce bias when
estimating the optimal value of a stochastic program. Our discussion focuses
on an asset allocation model with a power utility function. As we will
describe, estimating the optimal value of such a problem plays a key role in
establishing the quality of a candidate solution, and reducing bias improves
our ability to do so efficiently. We develop a jackknife estimator that is
adaptive in that it does not assume the order of the bias is known a priori.
![]()