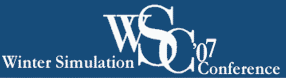 |
WSC 2007 Final Abstracts |
Monday 10:30:00 AM 12:00:00 PM
Tutorial: Monte Carlo Simulation in
Financial Engineering
Chair: Scott Nestler (University of Maryland)
Monte Carlo Simulation in Financial
Engineering
Nan Chen (Chinese University of Hong Kong) and L. Jeff
Hong (Hong Kong University of Science and Technology)
Abstract:
This paper reviews the use of Monte Carlo simulation in
the field of financial engineering. It focuses on several interesting topics
and introduces their recent development, including path generation, pricing
American-style derivatives, evaluating Greeks and estimating value-at-risk.
The paper is not intended to be comprehensive survey of the research
literature.
Monday 1:30:00 PM 3:00:00 PM
Risk Management and Sensitivity
Analysis
Chair: Kay Giesecke (Stanford
University)
Sensitivity Estimates From Characteristic
Functions
Paul Glasserman and Zongjian Liu (Columbia University)
Abstract:
The likelihood ratio method (LRM) estimates parameter
sensitivities by multiplying the output of a simulation by a random weight.
This weight is a differentiated log density, also called a score function. We
investigate the problem of computing LRM estimators when the relevant
densities are unknown but their characteristic functions are available, a
situation that arises in financial applications. We analyze the various
sources of error introduced when the score function is computed through
numerical transform inversion.
Kernel Estimation for Quantile
Sensitivities
Guangwu Liu and L. Jeff Hong (The Hong Kong
University of Science and Technology)
Abstract:
Quantiles, also known as value-at-risk in financial
applications, are important measures of random performance. Quantile
sensitivities provide information on how changes in the input parameters
affect the output quantiles. In this paper, we study the estimation of
quantile sensitivities using simulation. We propose a new estimator by
employing kernel method and show its consistency and asymptotic normality for
i.i.d. data. Numerical results show that our estimator works well for the test
problems.
A Confidence Interval for Tail Conditional
Expectation Via Two-level Simulation
Hai Lan, Barry L. Nelson, and
Jeremy Staum (Northwestern University)
Abstract:
We develop and evaluate a two-level simulation
procedure that produces a confidence interval for tail conditional
expectation, otherwise known as conditional tail expectation. This risk
measure is closely related to conditional value-at-risk, expected shortfall,
and worst conditional expectation. The outer level of simulation generates
risk factors and the inner level estimates each expected loss conditional on
the risk factor. Our procedure uses the statistical theory of empirical
likelihood to construct a confidence interval, and it uses tools from the
ranking-and-selection literature to make the simulation efficient.
Monday 3:30:00 PM 5:00:00 PM
Credit Risk
Chair: Nan Chen
(Chinese University of Hong Kong)
Efficient Monte Carlo Methods for Convex Risk
Measures in Portfolio Credit Risk Models
Joern Dunkel (Universitat
Augsburg) and Stefan Weber (Cornell University)
Abstract:
We discuss efficient Monte Carlo (MC) methods for the
estimation of convex risk measures within the portfolio credit risk model
CreditMetrics. Our focus lies on the Utility-based Shortfall Risk (SR)
measures, as these avoid several deficiencies of the current industry standard
Value-at-Risk (VaR). It is demonstrated that the importance sampling method
exponential twisting provides computationally efficient SR estimators.
Numerical simulations of test portfolios illustrate the good performance of
the proposed algorithms.
Estimating Tranche Spreads by Loss Process
Simulation
Kay Giesecke and Baeho Kim (Stanford University)
Abstract:
A credit derivative is a path dependent contingent
claim on the aggregate loss in a portfolio of credit sensitive securities. We
estimate the value of a credit derivative by Monte Carlo simulation of the
affine point process that models the loss. We consider two algorithms that
exploit the direct specification of the loss process in terms of an intensity.
One algorithm is based on the simulation of intensity paths. Here
discretization introduces bias into the results. The other algorithm
facilitates exact simulation of default times and generates an unbiased
estimator of the derivative price. We implement the algorithms to value index
and tranche swaps, and we calibrate the loss process to quotes on the CDX
North America High Yield index.
Approximations and Control Variates for Pricing
Portfolio Credit Derivatives
Zhiyong Chen (Bear, Stearns & Co.
Inc.) and Paul Glasserman (Columbia Business School)
Abstract:
Portfolio credit derivatives that depend on default
correlation are increasingly widespread in the credit market. Valuing such
products often entails Monte Carlo simulation. However, for large portfolios,
plain Monte Carlo simulation can be slow. In this paper, we develop
approximation methods for pricing collateralized debt obligation (CDO)
tranches in the widely used factor copula approach. We also discuss using the
approximations as control variates to improve the precision of Monte Carlo
estimates. These approximation methods and control variate techniques could be
applied to pricing other portfolio credit derivatives as well.
Tuesday 8:30:00 AM 10:00:00 AM
Derivative Security Pricing
Chair: Stefan Weber (Cornell University)
Efficient Estimation of Option Price and Price
Sensitivities via Structured Database Monte Carlo (SDMC)
Gang Zhao,
Tarik Borogovac, and Pirooz Vakili (Boston University)
Abstract:
We describe how to develop generic efficient simulation
algorithms for estimating price and price sensitivities (the Greeks) of
financial options using the Structured Database Monte Carlo (SDMC) approach.
These algorithms are based on stratification, control variate and a
combination of the two in an SDMC setting. Experimental results and some
discussion of the effectiveness of the approach are provided. The algorithms
also serve as illustrations of the basic approach of developing variance
reduction algorithms in an SDMC setting that are not necessarily limited to
stratification and control variate techniques.
American Option Pricing Under Stochastic
Volatility: A Simulation-based Approach
Arunachalam Chockalingam
(Purdue University) and Kumar Muthuraman (University of Texas)
Abstract:
We consider the problem of pricing American options
when the volatility of the underlying asset price is stochastic. No specific
stochastic volatility model is assumed for the stochastic process. We propose
a simulation-based approach to pricing such options. Iteratively, the method
determines the optimal exercise boundary and the associated price function for
a general stochastic volatility model. Given an initial guess of the optimal
exercise boundary, the Retrospective Approximation (RA) technique is used to
calculate the associated value function. Using this function, the exercise
boundary is improved and the process repeated till convergence. This method is
a simulation based variant of the exercise-policy improvement scheme developed
in Chockalingam and Muthuraman (2007). An illustration of the method is
provided when using the Heston (1993) model to represent the dynamics of the
volatility, together with comparisons against existing methods to validate our
numerical results.
Monte Carlo Methods for Valuation of Ratchet Equity
Indexed Annuities
Ming-hua Hsieh and Yu-fen Chiu (National Chengchi
University)
Abstract:
Equity Indexed Annuities (EIAs) are popular insurance
contracts. EIAs provide the insured with a guaranteed accumulation rate on
their premium at maturity. In addition, the insured may receive extra benefit
if the return of the linked index is high enough. There are a few variations
of EIAs. We consider two types of EIAs: compound ratchet and simple ratchet.
Under the geometric Brownian motion assumption for the equity index, plain
compound ratchet options is known to have closed form solutions, but plain
simple ratchet option is not. In this paper, we derive a closed form solution
for plain simple ratchet option. For more exotic options, Monte Carlo methods
are usually used for their valuation. To improve their efficiency, we propose
two control variates based on the analytical solutions for the price of plain
ratchet options. The effectiveness of the proposed control variates is
examined via numerical examples of a typical contract.
Tuesday 10:30:00 AM 12:00:00 PM
Portfolio Optimization
Chair:
Jeff Hong (Hong Kong University of Science and
Technology)
Non-Gaussian Asset Allocation in the Federal
Thrift Savings Plan
Scott T. Nestler (University of Maryland)
Abstract:
Historical data suggest that returns of stocks and
indices are not distributed independent and identically Normal, as is commonly
assumed. Instead, returns of financial assets are often skewed and have higher
kurtosis. In this study, we investigate how the optimal investment choices in
the federal government's Thrift Savings Plan (TSP) change when a non-Gaussian
factor model for returns, generated with independent components analysis (ICA)
and follow-ing the Variance Gamma (VG) process, is used in place of the usual
Normally-distributed returns model. Using back-testing and simulation, we hope
to show how this method could benefit the more than 3 million TSP participants
in achieving their retirement savings objectives.
Path-wise Estimators and Cross-path Regressions: An
Application to Evaluating Portfolio Strategies
Martin B. Haugh
(None) and Ashish Jain (Columbia Business School)
Abstract:
Recently developed dual techniques allow us to evaluate
a given sub-optimal dynamic portfolio policy by using the policy to construct
an upper bound on the optimal value function. Since it is easy to construct a
lower bound by simulating the given policy, we may use the distance from the
lower bound to the upper bound to assess the quality of the policy. One of the
difficulties that arises when computing the upper bound, however, is that we
need to know the sub-optimal policy's value function and its partial
derivatives with respect to all state variables. In this paper we show how
path-wise Monte-Carlo estimators together with the cross-path regression
approach can be used to estimate the sub-optimal value function and its
derivatives, thereby enabling us to compute the more theoretically satisfying
upper bound on the optimal value function.
An Empirical Comparison Between Nonlinear Programming
Optimization and Simulated Annealing (SA) Algorithm Under a Higher Moments
Bayesian Portfolio Selection Framework
Jingjing Lu and Merrill
Liechty (Drexel University)
Abstract:
The optimal portfolio selection problem has long been
of interest to both academics and practitioners. A higher moments Bayesian
portfolio optimization model can overcome the shortcomings of the traditional
Markowitz approach and take into consideration the skewness of asset returns
and parameter uncertainty. This paper presents a comparison between the
simulated annealing and the nonlinear programming methods of optimization for
the Bayesian portfolio selection problem in which the objective function
includes the portfolio mean, variance and skewness. We make the comparison for
a utility function that is easily optimized using both methods. In particular
we maximize a cubic utility function, and our results show that to achieve the
same level of accuracy, the CPU time for the nonlinear programming
optimization will be shorter than for the simulated annealing algorithm.
Though it is slower, the simulated annealing algorithm is still a viable
option for this utility function.
![]()