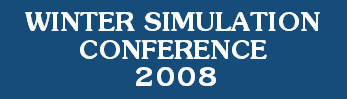 |
WSC 2008 Final Abstracts |
Tuesday 10:30:00 AM 12:00:00 PM
Tutorial on Monte Carlo Simulation of
Diffusions
Chair: Jose Blanchet (Columbia
University)
Monte Carlo Simulation of
Diffusions
Peter W Glynn (Stanford University)
Abstract:
This tutorial is intended to provide an overview of the
key algorithms that are used to simulate sample paths of diffusion processes,
as well as to offer an understanding of their fundamental approximation
properties.
Tuesday 1:30:00 PM 3:00:00 PM
Monte Carlo Methods in Credit Risk and
Sensitivity
Chair: Kay Giesecke (Stanford
University)
Simulating Point Processes by Intensity
Projection
Kay Giesecke, Hossein Kakavand, and Mohammad Mousavi
(Stanford University)
Abstract:
Point processes with stochastic intensities are
ubiquitous in many application areas, including finance, insurance,
reliability and queuing. They can be simulated from standard Poisson arrivals
by time-scaling with the cumulative intensity, whose path is typically
generated with a discretization method. However, discretization introduces
bias into the simulation results. This paper proposes a method for the exact
simulation of point processes with stochastic intensities. The method leads to
unbiased estimators. It is illustrated for a point process whose intensity
follows an affine jump-diffusion process.
Beta Approximations for Bridge
Sampling
Paul Glasserman and Kyoung-Kuk Kim (Columbia University,
Graduate School of Business)
Abstract:
We consider the problem of simulating X conditional on
the value of X+Y, when X and Y are independent positive random variables. We
propose approximate methods for sampling (X|X+Y) by approximating the fraction
(X/z|X+Y=z) with a beta random variable. We discuss applications to Levy
processes and infinitely divisible distributions, and we report numerical
tests for Poisson processes, tempered stable processes, and the Heston
stochastic volatility model.
Connecting the Top-Down to the Bottom-Up: Pricing CDO
under a Conditional Survival (CS) Model
Steven S. G. Kou and
Xianhua Peng (Columbia University)
Abstract:
In this paper, we use exact simulation to price CDO
under a new dynamic model, the Conditional Survival (CS) model, which provided
excellent calibration to both iTraxx tranches and underlying single name CDS
spreads on March 14, 2008, the day before the collapse of Bear Sterns, when
the market was highly volatile. The distinct features of the CS model include:
(1) it is able to generate clustering of defaults occurring dynamically in
time and strong cross-sectional correlation, i.e., the simultaneous defaults
of many names, both of which have been evidenced in the current subprime
mortgage crisis; (2) it incorporates idiosyncratic default risk of single
names but does not specify concrete models for them; (3) it provides automatic
calibration to underlying single name CDS; (4) it allows fast CDO tranche
pricing and calculation of sensitivity of CDO tranches to underlying single
name CDS.
Tuesday 3:30:00 PM 5:00:00 PM
Variance Reduction in Risk Analysis
Chair: Ming-hua Hsieh (National Chengchi
University)
Reducing the Variance of Likelihood Ratio Greeks
in Monte Carlo
Luca Capriotti (Global Modelling and Analytics
Group, Credit Suisse Investment Banking Division)
Abstract:
We investigate the use of Antithetic Variables, Control
Variates and Importance Sampling to reduce the statistical errors of option
sensitivities calculated with the Likelihood Ratio Method in Monte Carlo. We
show how Antithetic Variables solve the well-known problem of the divergence
of the variance of Delta for short maturities and small volatilities. With
numerical examples within a Gaussian Copula framework, we show how simple
Control Variates and Importance Sampling strategies provide computational
savings up to several orders of magnitude.
Revisit of Stochastic Mesh Method for Pricing
American Options
Guangwu Liu and Jeff Hong (Hong Kong University of
Science and Technology)
Abstract:
We revisit the stochastic mesh method for pricing
American options, from a conditioning viewpoint, rather than the importance
sampling viewpoint of Broadie and Glasserman (1997). Starting from this new
viewpoint, we derive the weights proposed by Broadie and Glasserman (1997) and
show that their weights at each exercise date use only the information of the
next exercise date (therefore, we call them forward-looking weights). We also
derive new weights that exploit not only the information of the next exercise
date but also the information of the last exercise date (therefore, we call
them binocular weights). We show how to apply the binocular weights to the
Black-Scholes model, more general diffusion models, and the variance-gamma
model. We demonstrate the performance of the binocular weights and compare to
the performance of the forward-looking weights through numerical experiments.
Valuation of Variable Annuity Contracts with
Cliquet Options in Asia Markets
Ming-hua Hsieh (National Chengchi
University)
Abstract:
Variable annuities are very appealing to the investor.
For example, in United States, sales volume on variable annuities grew to a
record 184 billion in calendar year 2006. However, due to their complicated
payoff structure, their valuation and risk management are challenges to the
insurers. In this paper, we study a variable annuity contract with cliquet
options in Asia markets. The contact has quanto feature. We propose an
efficient Monte Carlo method to value the contract. Numerical examples suggest
our approach is quite efficient.
Wednesday 8:30:00 AM 10:00:00 AM
Efficient Monte Carlo Methods for
Risk Measures
Chair: Soumyadip Ghosh (IBM TJ Watson Research Center)
Efficient Tail Estimation for Sums of Correlated
Lognormals
Jose Blanchet (Columbia University), Sandeep Juneja
(Tata Institute of Fundamental Research) and Leonardo Rojas-Nandayapa
(Univeristy of Aarhus)
Abstract:
Our focus is on efficient estimation of tail
probabilities of sums of correlated lognormals. This problem is motivated by
the tail analysis of portfolios of assets driven by correlated Black-Scholes
models. We propose three different procedures that can be rigorously shown to
be asymptotically optimal as the tail probability of interest decreases to
zero. The first algorithm is based on importance sampling and is as easy to
implement as crude Monte Carlo. The second algorithm is based on an elegant
conditional Monte Carlo strategy which involves polar coordinates and the
third one is an importance sampling algorithm that can be shown to be strongly
efficient.
A Rate Result for Simulation Optimization with
Conditional Value-at-Risk Constraints
Soumyadip Ghosh (IBM TJ
Watson Research Center)
Abstract:
We study a stochastic optimization problem that has its
roots in financial portfolio design. The problem has a specified deterministic
objective function and constraints on the conditional value-at-risk of the
portfolio. Approximate optimal solutions to this problem are usually obtained
by solving a sample-average approximation. We derive bounds on the gap in the
objective value between the true optimal and an approximate solution so
obtained. We show that under certain regularity conditions the approximate
optimal value converges to the true optimal at the canonical rate
O(n-1/2), where n represents the sample size. The
constants in the expression are explicitly defined.
Optimizing Portfolio Tail Measures: Asymptotics and
Efficient Simulation Optimization
Sandeep Juneja (Tata Institute of
Fundamental Research)
Abstract:
We consider a portfolio allocation problem where the
objective function is a tail event such as probability of large portfolio
losses. The dependence between assets is captured through multi-factor linear
model. We address this optimization problem using two broad approaches. We
show that a suitably scaled asymptotic of the probability of large losses can
be developed that is a simple convex function of the allocated resources.
Thus, asymptotically, portfolio allocation problem is approximated by a convex
programming problem whose solution is easily computed and provides significant
managerial insight. We then solve the original problem using sample average
simulation optimization. Since rare events are involved, naive simulation may
perform poorly. To remedy this, we introduce change-of-variable based
importance sampling technique and develop a single change of measure that
asymptotically optimally estimates tail probabilities across the entire space
of feasible allocations.
Wednesday 10:30:00 AM 12:00:00 PM
Monte Carlo Risk Analysis in
Finance, Operations, and Optimization
Chair: Xianzhe Chen (North Dakota
State University)
Response Surface Methodology for Simulating Hedging
and Trading Strategies
Evren Baysal, Barry L. Nelson, and Jeremy
Staum (Northwestern University)
Abstract:
Suppose that one wishes to evaluate the distribution of
profit and loss (P&L) resulting from a dynamic trading strategy. A
straightforward method is to simulate thousands of paths (i.e., time series)
of relevant financial variables and to track the resulting P&L at every
time at which the trading strategy rebalances its portfolio. In many cases,
this requires numerical computation of portfolio weights at every rebalancing
time on every path, for example, by a nested simulation performed conditional
on market conditions at that time on that path. Such a two-level simulation
could involve many millions of simulations to compute portfolio weights, and
thus be too computationally expensive to attain high accuracy. We show that
response surface methodology enables a more efficient simulation procedure: in
particular, it is possible to do far fewer simulations by using kriging to
model portfolio weights as a function of underlying financial variables.
Supply Chain Risks Analysis by Using Jump-Diffusion
Model
Xianzhe Chen and Jun Zhang (North Dakota State University)
Abstract:
This paper investigates the effects of demand risk on
the performance of supply chain in continuous time setting. The inventory
level has been modeled as a jump-diffusion process and the two-number
inventory policy has been implemented in the supply chain system. The
simulated annealing algorithm has been used to search the optimal critical
values of the two-number policy. The jump magnitude has been considered in two
cases: constant and Laplace distribution which has favorable property, i.e.
leptokurtic. Numerical studies have been conducted for various scenarios to
provide insights of effects of demand disruption on the performance of the
supply chain.
A Particle Filtering Framework for Randomized
Optimization Algorithms
Enlu Zhou, Michael C. Fu, and Steven I.
Marcus (University of Maryland, College Park)
Abstract:
We propose a framework for optimization problems based
on particle filtering (also called Sequential Monte Carlo method). This
framework unifies and provides new insight into randomized optimization
algorithms. The framework also sheds light on developing new optimization
algorithms through the freedom in the framework and the various improving
techniques for particle filtering.
![]()