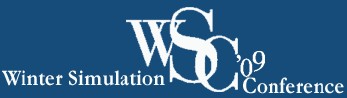 |
WSC 2009 Final Abstracts
|
Methodology - Analysis Methodology Track
Monday 10:30:00 AM 12:00:00 PM
Learning Based Analysis
Chair: Shane Henderson (Cornell University)
Strategic Analysis with Simulation-based Games
Yevgeniy Vorobeychik (University of Pennsylvania) and Michael Wellman (University of Michigan)
Abstract:
We present an overview of an emerging methodology for applying game-theoretic analysis to strategic environments described by a simulator. We ï¬rst introduce the problem of solving a simulation-based game, and proceed to review convergence results and confidence bounds about game-theoretic equilibrium estimates. Next, we present techniques for approximating equilibria in simulation-based games, and close with a series of applications.
Reinforcement Learning for Model Building and Variance-Penalized Control
Abhijit Gosavi (Missouri University of Science and Technology)
Abstract:
Reinforcement learning (RL) is a simulation-based technique to solve Markov decision problems or processes (MDPs). It is especially useful if the transition probabilities in the MDP are hard to find
or if the number of states in the problem is too large. In this paper, we present a new model-based RL algorithm that builds the transition probability model without the generation of the transition probabilities; the literature on model-based RL attempts
to compute the transition probabilities. We also present a variance-penalized Bellman equation and an RL algorithm that uses it
to solve a variance-penalized MDP. We conclude with some numerical experiments with these algorithms.
Estimating the Probability That the Game of Monoply Never Ends
Eric J. Friedman, Shane G. Henderson, Thomas Byuen, and German Gutierrez Gallardo (Cornell University)
Abstract:
We estimate the probability that the game of Monopoly between two players playing very simple strategies never ends. Four different estimators, based respectively on straightforward simulation, a Brownian motion approximation, asymptotics for Markov chains, and importance sampling all yield an estimate of approximately twelve percent.
Monday 10:30:00 AM 12:00:00 PM
Stochastic Modeling
Chair: Pierre L'Ecuyer (University of Montreal)
Simulation Model Calibration with Correlated Knowledge-Gradients
Peter Frazier, Warren Buckler Powell, and Hugo Simao (Princeton University)
Abstract:
We address the problem of calibrating an approximate dynamic programming model, where we need to find a vector of parameters to produce the best fit of the model against historical data. The problem requires adaptively choosing the sequence of parameter settings on which to run the model, where each run of the model requires approximately twelve hours of CPU time and produces noisy non-stationary output. We describe an application of the knowledge-gradient algorithm with correlated beliefs to this problem and show that this algorithm finds a good parameter vector out of a population of one thousand with only three runs of the model.
Fitting A Normal Copula For A Multivariate Distribution With Both Discrete And Continuous Marginals
Nabil Channouf (GERAD) and Pierre L'Ecuyer (University of Montreal)
Abstract:
We consider a multivariate distribution with both discrete and continuous marginals, for which the dependence is modeled by a normal copula sometimes called the NORTA method), and provide an algorithm for fitting the copula in that situation. The fitting is done by matching (approximately) either the rank correlations or the product moment correlations for all pairs of marginals. Numerical illustrations are provided.
Monday 1:30:00 PM 3:00:00 PM
Large Deviation
Chair: Jose Blanchet (Columbia University)
Do Mean-based Ranking and Selection Procedures Consider Systems' Risk?
Demet Batur (University of Nebraska-Lincoln) and Fred Choobineh (IMSE, University of Nebraska-Lincoln)
Abstract:
The legacy simulation approach in ranking and selection procedures compares systems based on a mean performance metric. The best system is most often deemed as the one with the largest (or smallest) mean performance metric. In this paper, we discuss the limitations of the mean-based selection approach. We explore other selection criterion and discuss new approaches based on stochastic dominance using an appropriate section of the distribution function of the performance metric. In this approach, the decision maker has the flexibility to determine a section of the distribution function based on the specific features of the selection problem representing either the downside risk, upside risk, or central tendency of the performance metric. We discuss two different ranking and selection procedures based on this new approach followed by a small experiment and present some open research problems.
Nested Simulation for Estimating Large Losses Within a Time Horizon
Sandeep Juneja (Tata Institute of Fundamental Research) and L Ramprasath (Institute for Financial Management and Research)
Abstract:
We consider the problem of estimating the probability that a stochastic process observed at discrete time intervals exceeds a specified threshold. We further assume that the value of this process at any time, along any realization, is a conditional expectation which is not known analytically but can be estimated via simulation. This leads to a nested simulation procedure. One application of this arises in risk management, where our interest may be in the probability that a portfolio exceeds a threshold of losses at specified times. Here, if the portfolio consists of sophisticated derivatives, then as a function of the underlying security prices, the portfolio value at any time is a conditional expectation that may be evaluated via simulation. In our analysis, we use the fact that conditional on the outer loop of the simulation, our estimation problem is related to the large deviations based ordinal optimization framework.
Efficient Rare Event Simulation of Continuous Time Markovian Perpetuities
Jose H. Blanchet (Columbia University) and Peter W. Glynn (Stanford University)
Abstract:
We develop rare event simulation methodology for the tail of a perpetuity driven by a continuous time Markov chain. We present a state dependent importance sampling estimator in continuous time that can be shown to be asymptotically optimal in the context of small interest rates.
Monday 1:30:00 PM 3:00:00 PM
Estimation and Sampling
Chair: Jeff Hong (The Hong Kong University of Science and Technology)
On the Error Distribution for Randomly-shifted Lattice Rules
Pierre L'Ecuyer (Université de Montréal) and Bruno Tuffin (INRIA - Rennes)
Abstract:
Randomized quasi-Monte Carlo (RQMC) methods estimate the expectation of a random variable by the average of n dependent realizations. Because of the dependence, the estimation error may not obey a central limit theorem. Analysis of RQMC methods have so far focused mostly on the convergence rates of asymptotic worst-case error bounds and variance bounds, when n increases, but little is known about the limiting distribution of the error. We examine this limiting distribution for the special case of a randomly-shifted lattice rule, when the integrand is smooth. We start with simple one-dimensional functions, where we show that the limiting distribution is uniform over a bounded interval if the integrand is non-periodic, and has a square root form over a bounded interval if the integrand is periodic. In higher dimensions, for linear functions, the distribution function of the properly standardized error converges to a spline of degree equal to the dimension.
Sampling Distribution of the Variance
Pierre L. Douillet (ENSAIT)
Abstract:
Without confidence intervals, any simulation is worthless. These intervals are quite ever obtained from the so called "sampling variance". In this paper, some well-known results concerning the sampling distribution of the variance are recalled and completed by simulations and new results. The conclusion is that, except from normally distributed populations, this distribution is more difficult to catch than ordinary stated in application papers.
A General Framework of Importance Sampling for Value-at-risk and Conditional Value-at-risk
Lihua Sun and Jeff Hong (The Hong Kong University of Science and Technology)
Abstract:
Value-at-risk (VaR) and conditional value-at-risk (CVaR) are important risk measures. Importance sampling (IS) is often used to estimate them. We derive the asymptotic representations for IS estimators of VaR and CVaR. Based on these representations, we are able to give simple conditions under which the IS estimators have smaller asymptotic variances than the ordinal estimators. We show that the exponential twisting can yield an IS distribution that satisfies the conditions for both the IS estimators of VaR and CVaR. Therefore, we may be able to estimate VaR and CVaR accurately at the same time.
Monday 3:30:00 PM 5:00:00 PM
Fitting Correlated Processes
Chair: Min-Hua Hsieh (National Chengchi University)
Fitting Discrete Multivariate Distributions With Unbounded Marginals And Normal-Copula Dependence
Athanassios N Avramidis (University of Southampton)
Abstract:
In specifying a multivariate discrete distribution via the NORTA method,
a problem of interest is:
given two discrete unbounded marginals and a target value $r$, find the correlation of the bivariate Gaussian copula that
induces rank correlation $r$ between these marginals.
By solving the analogous problem with the marginals replaced
by finite-support (truncated) counterparts, an approximate solution can be obtained.
Our main contribution is an upper bound on the absolute error,
where error is defined as the difference between $r$ and
the resulting rank correlation between the original unbounded marginals.
Furthermore, we propose a simple method for truncating the support while
controlling the error via the bound, which is a sum of scaled squared tail probabilities.
Examples where both marginals are discrete Pareto demonstrate considerable
work savings against an alternative simple-minded truncation.
On the Performance of the Cross-Entropy Method
Jiaqiao Hu and Ping Hu (State University of New York, Stony Brook)
Abstract:
We study the recently introduced Cross-Entropy (CE) method for optimization, an iterative random sampling approach that is based on sampling and updating an underlying distribution function over the set of feasible solutions. In particular, we propose a systematic approach to investigate the convergence and asymptotic convergence rate for the CE method through a novel connection with the well-known stochastic approximation procedures. Extensions of the approach to stochastic optimization will also be discussed.
New Estimators for Parallel Steady-state Simulations
Ming-hua Hsieh (National Chengchi University, TAIWAN) and Peter W. Glynn (Stanford University)
Abstract:
When estimating steady-state parameters in parallel discrete event
simulation, initial transient is an important issue to consider. To
mitigate the impact of initial condition on the quality of the
estimator, we consider a class of estimators obtained by
putting different weights on the sampling average across
replications at selected time points. The weights are chosen to
maximize their Gaussian likelihood.
Then we apply model selection criterion due to Akaike and Schwarz to select two of them as our proposed estimators.
In terms of relative root MSE, the proposed estimators compared favorably to
the standard time average estimator in a typical test problem with significant initial transient.
Monday 3:30:00 PM 5:00:00 PM
Simulation Estimation
Chair: David Morton (The University of Texas at Austin)
Bayesian Non-Parametric Simulation of Hazard Functions
Dmitriy Belyi, Paul Damien, David Morton, and Elmira Popova (The University of Texas at Austin)
Abstract:
In Bayesian non-parametric statistics, the extended gamma process can be used to model the class of monotonic hazard functions. However, numerical evaluations of the posterior process are very difficult to compute for realistic sample sizes. To overcome this, we use Monte Carlo methods introduced by Laud, Smith, and Damien (1996) to simulate from the posterior process. We show how these methods can be used to approximate the increasing failure rate of an item, given observed failures and censored times. We then use the results to compute the optimal maintenance schedule under a specified maintenance policy.
Simulating Cointegrated Time Series
Alexander Galenko (PENSON Financial Services), David Morton and Elmira Popova (The University of Texas at Austin) and Ivilina Popova (Texas State University)
Abstract:
When one models dependence solely via correlations, portfolio allocation models can perform poorly. This motivates considering dependence measures other than correlation. Cointegration is one such measure that captures long-term dependence. In this paper we present a new method to simulate cointegrated sample paths using the vector auto-regressive-to-anything (VARTA) algorithm. Our approach relies on new properties of cointegrated time series of financial asset prices and allows for marginal distributions from the Johnson system. The method is illustrated on two data sets, one real and one artificial.
Estimating the Efficient Frontier of a Probabilistic Bicriteria Model
Tara Rengarajan and David P. Morton (The University of Texas at Austin)
Abstract:
We consider a problem that trades off cost of a system design with the risk of that design, where risk is measured by the probability of a bad event, such as system failure. Our interest lies in the problem class where we cannot evaluate this risk measure exactly. We approach this problem via a bicriteria optimization model, replacing the risk measure by an Monte Carlo estimator and solving a parametric family of optimization models to produce an approximate efficient frontier. Optimizing system design with the risk estimator requires solving a mixed integer program. We show we can minimize risk over a range of cost thresholds or minimize cost over a range of risk thresholds and examine associated asymptotics. The proximity of the approximate efficient frontier to the true efficient frontier is established via an asymptotically valid confidence interval with minimal additional work. Our approach is illustrated using a facility-sizing problem.
Tuesday 8:30:00 AM 10:00:00 AM
Ranking and Selection
Chair: Steve Chick (INSEAD)
Adapt Selection Procedures to Process Correlated and Non-Normal Data with Batch Means
E Jack Chen (BASF Corporation)
Abstract:
Many simulation output analysis procedures are derived based on the assumption that data are independent and identically
distributed (I.I.D.) normal; examples include ranking and selection procedures and multiple-comparison procedures. The method of batch means is the technique of choice to manufacture data that are approximately I.I.D. normal when
the raw samples are not. Batch means are sample means of subsets of consecutive subsamples from a simulation output sequence. We propose to incorporate the procedure of determining the batch
size to obtain approximately I.I.D. normal batch means into the selection procedures of comparing the performance of alternative
system designs. We performed an empirical study to evaluate the performance of the
extended selection procedure.
Statistical Analysis and Comparison of Simulation Models of Highly Dependable Systems Models of Highly Dependable Systems - an Experimental Study
Peter Buchholz and Dennis Müller (TU Dortmund)
Abstract:
The validation of dependability or performance requirements is often done
experimentally using simulation experiments. In several applications, the
experiments have a binary output which describes whether a requirement is met
or not. In highly dependable systems the probability of missing a requirement
is 10^{-6} or below which implies that statistically significant results have to be
computed for binomial distributions with a small probability.
In this paper we compare different methods to statistically evaluate simulation experiments with highly dependable systems. Some of the available methods are extended slightly to handle small probabilities and large samples
sizes. Different problems like the computation
of one or two sided confidence intervals, the comparison of different systems and the ranking of systems are considered.
The Conjunction of the Knowledge Gradient and the Economic Approach to Simulation Selection
Stephen E. Chick (INSEAD) and Peter Frazier (Princeton University)
Abstract:
This paper deals with the selection of the best of a finite set of
systems, where best is defined with respect to the maximum mean
simulated performance. We extend the ideas of the knowledge
gradient, which accounts for the expected value of one stage of
simulation, by accounting for the future value of the option to
simulate over multiple stages. We extend recent work on the
economics of simulation, which studied discounted rewards, by
balancing undiscounted simulation costs and the expected value of
information from simulation runs. This contribution results in a
diffusion model for comparing a single simulated system with a
standard that has a known expected reward, and new stopping rules
for fully sequential procedures when there are multiple systems.
These stopping rules are more closely aligned with the expected
opportunity cost allocations that are effective in numerical tests.
We demonstrate an improvement in performance over previous methods.
Tuesday 10:30:00 AM 12:00:00 PM
Simulation Optimization Using Metamodels
Chair: Hong Wan (Purdue University)
Robust Simulation-Optimization Using Metamodels
Gabriella Dellino (University of Siena), Jack P.C. Kleijnen (Tilburg University) and Carlo Meloni (Polytechnic of Bari)
Abstract:
Optimization of simulated systems is the goal of many methods, but most methods assume known environments. In this
paper we present a methodology that does account for uncertain environments. Our methodology uses Taguchi�s view of the
uncertain world, but replaces his statistical techniques by either Response Surface Methodology or Kriging metamodeling.
We illustrate the resulting methodology through the well-known Economic Order Quantity (EOQ) model.
Simulation Optimization with Hybrid Golden Region Search
Alireza Kabirian (University of Alaska-Anchorage) and Sigurdur Olafsson (Iowa State University)
Abstract:
Simulation Optimization (SO) is a class of mathematical optimization techniques in which the objective function could only be numerically evaluated through simulation. In this paper, a new SO approach called Golden Region (GR) search is developed for continuous problems. GR divides the feasible region into a number of (sub) regions and selects one region in each iteration for further search based on the quality and distribution of simulated points in the feasible region and the result of scanning the response surface through a metamodel. The experiments show the GR method is efficient compared to three well-established approaches in the literature. We also prove the convergence in probability to global optimum for a large class of random search methods in general and GR in particular.
Stochastic Trust Region Response Surface Convergent Method for Generally Distributed Response Surface
Kuohao Chang (National Tsing Hua University) and Hong Wan (Purdue University)
Abstract:
Simulation optimization refers to the iterative procedure in search of the optimal parameter when the objective function can only be evaluated by stochastic simulation. STRONG (Stochastic Trust Region Response Surface Convergent Method) is a newly developed design-of-experiments based simulation optimization method. It
incorporates the idea of trust region method (TRM) for deterministic optimization into the traditional response surface methodology (RSM) to eliminate the human intervention required by RSM and to achieve the desired convergence. In the earlier paper, we proved the convergence of STRONG and demonstrated its computational efficiency. The original STRONG assumes the stochastic response follows a normal
distribution. This paper relaxes the normal assumption and develops a framework called STRONG-X which is applicable for generally distributed additive noise with bounded variance. The convergence of STRONG-X can be proved and the generality of STRONG-X makes it an
appealing method.
Tuesday 1:30:00 PM 3:00:00 PM
Optimal Computing Budget Allocation
Chair: Douglas Morrice (The University of Texas at Austin)
Selection of the Best with Stochastic Constraints
Alireza Kabirian (University of Alaska-Anchorage) and Sigurdur Olafsson (Iowa State University)
Abstract:
When selecting the best design of a system among a finite set of possible designs, there may be multiple selection criterion. One formulation of such a multi-criteria problem is minimization (or maximization) of one of the criterions while constrain-ing the others. In this paper, we assume the criteria are unobservable mean values of stochastic outputs of simulation. We propose a new heuristic iterative algorithm for finding the best in this situation and use a number of experiments to demon-strate the performance of the algorithm.
Optimal Computing Budget Allocation for Constrained Optimization
Nugroho Artadi Pujowidianto and Loo Hay Lee (National University of Singapore), Chun-Hung Chen (George Mason University) and Chee Meng Yap (National University of Singapore)
Abstract:
In this paper, we consider the problem of selecting the best design from a discrete number of alternatives in the presence of a stochastic constraint via simulation experiments. The best design is the design with smallest mean of main objective among the feasible designs. The feasible designs are the designs of which constraint measure is below the constraint limit. The Optimal Computing Budget Allocation (OCBA) framework is used to tackle the problem. In this framework, we aim at maximizing the probability of correct selection given a computing budget by controlling the number of simulation replications. An asymptotically optimal allocation rule is derived. A comparison with Equal Allocation (EA) in the numerical experiments shows that the proposed allocation rule gains higher probability of correct selection.
A Transient Means Ranking and Selection Procedure with Sequential Sampling Constraints
Douglas J. Morrice (The University of Texas at Austin) and Mark W. Brantley and Chung-Hung Chen (George Mason University)
Abstract:
We develop a Ranking and Selection procedure for selecting the best configuration based on a transient mean performance measure. The procedure extends the OCBA approach to systems whose means are a function of some other variable such as time. In particular, we characterize this as a prediction problem and imbed a regression model in the OCBA procedure. In this paper, we analyze a problem with sequential sampling constraints for each configuration and offer a heuristic to use a poly-nomial regression model when variance reduction is possible.
Tuesday 3:30:00 PM 5:00:00 PM
Simulation Optimization
Chair: Kim Sujin (National University of Singapore)
An Adaptive Multidimensional Version of the Kiefer-Wolfowitz Stochastic Approximation Algorithm
Mark Broadie, Deniz M Cicek, and Assaf Zeevi (Columbia Business School)
Abstract:
We extend the scaled-and-shifted Kiefer-Wolfowitz (SSKW) algorithm developed by Broadie, Cicek, and Zeevi (2009) to
multiple dimensions. The salient feature of this algorithm is that it makes adjustments of the tuning parameters that adapt to
the underlying problem characteristics. We compare the performance of this algorithm to the traditional Kiefer-Wolfowitz
(KW) one and observe significant improvement in the finite-time behavior on some stylized test functions and a
multidimensional newsvendor problem.
Newton-Raphson Version of Stochastic Approximation over Discrete Sets
Eunji Lim (University of Miami)
Abstract:
This paper considers the problem of optimizing a complex stochastic system over a discrete set of feasible values of a parameter when the objective function can only be estimated through simulation. We propose a new gradient-based method that mimics the Newton-Raphson method and makes use of both the gradient and the Hessian of the objective function. The proposed algorithm is designed to give guidance on how to choose the sequence of gains which plays a critical role in the empirical performance of a gradient-based algorithm. In addition to the desired fast convergence in the first few steps of the procedure, the proposed algorithm converges to a local optimizer with probability one as n goes to infinity with rate 1/n where n is the number of iterations.
Pareto Front Approximation with Adaptive Weighted Sum Method in Multiobjective Simulation Optimization
Jong-hyun Ryu (Purdue University), Sujin Kim (National University of Singapore) and Hong Wan (Purdue University)
Abstract:
This work proposes a new method for approximating Pareto front of a multi-objective simulation optimization problem
(MOP) where the explicit forms of the objective functions are not available. The method iteratively approximates each
objective function using a metamodeling scheme and employs a weighted sum method to convert the MOP into a set of
single objective optimization problems. The weight on each single objective function is adaptively determined by accessing
newly introduced points at the current iteration and the non-dominated points so far. A trust region algorithm is applied to
the single objective problems to search for the points on the Pareto front. The numerical results show that the proposed
algorithm efficiently generates evenly distributed points for various types of Pareto fronts.
Wednesday 8:30:00 AM 10:00:00 AM
Metamodel and Simulation Modeling
Chair: Szu Ng (National University of Singapore)
G-SSASC: Simultaneous Simulation of System Models with Bounded Hazard Rates
Shravan Gaonkar and William H. Sanders (University of Illinois, Urbana Champaign)
Abstract:
The real utility of simulation lies in comparing different design
choices by evaluating models represented using a simulation framework.
In an earlier paper, we presented the Simultaneous Simulation of
Alternative System Configurations (SSASC) simulation algorithm,
which provides a methodology to
exploit the structural/stochastic similarity among the alternative
design configurations in an efficient manner that evaluates multiple alternative
configurations of a system design simultaneously.
However, this technique was limited to Markovian models.
In this paper, we propose GSSASC, which expands the domain of system models
that can be modeled and evaluated to those non-Markovian models that have
distributions with bounded hazard rates.
We also show that we obtain a speed-up of up to an order of magnitude for a
case study model that evaluates the reliability of a storage system.
A Study on the Effects of Parameter Estimation on Kriging Model's Prediction Error in Stochastic Simulations
Jun Yin, Szu Hui Ng, and Kien Ming Ng (National University of Singapore)
Abstract:
In the application of kriging model in the field of simulation, the parameters of the model are likely to be estimated from the simulated data. This introduces parameter estimation uncertainties into the overall prediction error, and this uncertainty can be further aggravated by random noise in stochastic simulations. In this paper, we study the effects of stochastic noise on parameter estimation and the overall prediction error. A two-point tractable problem and three numerical experiments are provided to show that the random noise in stochastic simulations can increase the parameter estimation uncertainties and the overall prediction error. Among the three kriging model forms studied in this paper, the modified nugget effect model captures well the various components of uncertainty and has the best performance in terms of the overall prediction error.
Wednesday 8:30:00 AM 10:00:00 AM
Output Analysis
Chair: James Wilson (North Carolina State University)
A Comparison of Markovian Arrival and ARMA/ARTA Processes for the Modeling of Correlated Input Processes
Falko Bause, Peter Buchholz, and Jan Kriege (TU Dortmund)
Abstract:
The adequate modeling of input processes often requires that correlation is taken into account and is a key issue in building realistic simulation models. In analytical modeling Markovian Arrival Processes (MAPs) are commonly used to describe correlated arrivals, whereas for simulation often ARMA/ARTA-based models are in use. Determining the parameters for the latter input models is well-known whereas good fitting methods for MAPs have been developed only in recent years. Since MAPs may as well be used in simulation models, it is natural to compare them with ARMA/ARTA models according to their expressiveness and modeling capabilities for dependent sequences. In this paper we experimentally compare MAPs and ARMA/ARTA-based models.
Omitting Meaningless Digits: Analyzing Ldr(1), the Standard Leading-digit Rule
Wheyming Tina Song (Tsing Hua University) and Bruce Schmeiser (Purdue Unviesity)
Abstract:
The standard leading-digit rule, LDR(1) is to omit point-estimator
digits to the right of the leading digit of the point-estimator's
standard error. Assuming that the original point estimator is
normally distributed, the authors previously showed that LDR(1)
guarantees---for all means and for all standard errors---that the
truncated estimator's first omitted digit is correct with
probability no greater than 0.117, not much greater than the 1-in-10
chance for a random digit. We consider two variations of the
previously studied LDR(1) truncated point estimator The first is
the truncated estimator with an implied appended digit ''5''. The
second is the rounded estimator, which truncates after appending the
''5''. Both point estimators have nearly identical statistical
properties, including negligible bias. In terms of root mean
squared error and in terms of correlation with the original
estimator, we establish here that the worst-case LDR(1) degradation
is about four percent.
N-Skart: A Nonsequential Skewness- and Autoregression-adjusted Batch-means Procedure for Simulation Analysis
Ali Tafazzoli (Metron Aviation, Inc.) and James R. Wilson (North Carolina State University)
Abstract:
We discuss N-Skart, a nonsequential procedure designed to deliver a
confidence interval (CI) for the steady-state mean of a simulation output
process when the user supplies a single simulation-generated time series
of arbitrary size and specifies the required coverage probability for a CI
based on that data set. N-Skart is a variant of the method of batch means
that exploits separate adjustments to the half-length of the CI so as to
account for the effects on the distribution of the underlying Student's
t-statistic that arise from skewness (nonnormality) and autocorrelation
of the batch means. If the sample size is sufficiently large, then N-Skart
delivers not only a CI but also a point estimator for the steady-state
mean that is approximately free of initialization bias. In an
experimental performance evaluation involving a wide range of test
processes and sample sizes, N-Skart exhibited close conformance to the
user-specified CI coverage probabilities.
Wednesday 10:30:00 AM 12:00:00 PM
Sequential Selection
Chair: Marvin Nakayama (New Jersey Institute of Technology)
A General Framework for the Asymptotic Validity of Two-Stage Procedures for Selection and Multiple Comparisons with Consistent Variance Estimators
Marvin K Nakayama (New Jersey Institute of Technology)
Abstract:
We consider two-stage procedures
for selection and multiple comparisons,
where the variance parameter is
estimated consistently.
We examine conditions under which
the procedures are asymptotically valid
in a general framework.
Our proofs of asymptotic validity require
that the estimators at the end
of the second stage are asymptotically
normal, so we require a random-time-change
central limit theorem.
We explain how the assumptions hold
for comparing means in transient simulations,
steady-state simulations
and quantile estimation,
but the assumptions are also valid
for many other problems
arising in simulation studies.
Analysis of Sequential Stopping Rules
Dashi I. Singham and Lee W. Schruben (University of California, Berkeley)
Abstract:
Sequential stopping rules applied to confidence interval procedures (CIPs) may lead to coverage that is less than nominal. This paper introduces a method for estimating coverage functions analytically in order to evaluate the potential loss of coverage. This method also provides an estimate for the distribution of the stopping time of the procedure. Knowledge of coverage functions could help evaluate and compare confidence interval procedures while avoiding lengthy empirical testing. Numerical implementation of our method shows that analytical coverage functions approximate those calculated empirically. Analytical coverage functions can be used to explain why many sequential procedures do not provide adequate coverage.
A Novel Sequential Design Strategy for Global Surrogate Modeling
Karel Crombecq (University of Antwerp), Dirk Gorissen (Ghent University), Luciano De Tommasi (University of Antwerp) and Tom Dhaene (Ghent University)
Abstract:
In mathematical/statistical modeling of complex systems, the locations of the data points are essential to the success of the algorithm. Sequential design methods are iterative algorithms that use data acquired from previous iterations to guide future sample selection. They are often used to improve an initial design such as a Latin hypercube or a simple grid, in order to focus on highly dynamic parts of the design space. In this paper, a comparison is made between different sequential design methods for global surrogate modeling on a real-world electronics problem. Existing exploitation and exploration-based methods are compared against a novel hybrid technique which incorporates both an exploitation criterion, using local linear approximations of the objective function, and an exploration criterion, using a Monte Carlo Voronoi tessellation. The test results indicate that a considerable improvement of the average model accuracy can be achieved by using this new approach.
Wednesday 10:30:00 AM 12:00:00 PM
Simulation Analysis
Chair: Jamie Weiland (Illinois State University)
Simulation Fusion
Wai Kin (Victor) Chan (Rensselaer Polytechnic Institute), Lee W. Schruben (University of California - Berkeley), Barry L. Nelson (Northwestern University) and Sheldon H. Jacobson (University of Illinois)
Abstract:
The concept of data fusion (DF) has had a major impact on statistical methodology and practice within the US Department of Defense. In this paper we explore expanding this idea to include simulation modeling and analysis. Simulation fusion (SF) is the concept of combining data, models, experiments, and analyses to improve the outcome of simulation studies. In this paper, we propose an initial framework and discuss various SF schemes. We provide several examples to illustrate these ideas and discuss some of the potential benefits of simulation fusion.
Influence Diagrams in Analysis of Discrete Event Simulation Data
Jirka Poropudas and Kai Matti Virtanen (Systems Analysis Laboratory, Helsinki University of Technology)
Abstract:
In this paper, influence diagrams (IDs) are used as simulation
metamodels to aid simulation based decision making. A decision
problem under consideration is studied using discrete event
simulation with decision alternatives as simulation parameters. The
simulation data are used to construct an ID that presents the
changes in simulation state with chance nodes. The decision
alternatives and objectives of the decision problem are included in
the ID as decision and utility nodes. The solution of the ID gives
the optimal decision alternatives, i.e., the values of the
simulation parameters that, e.g., maximize the expected value of the
utility function measuring the attainment of the objectives.
Furthermore, the constructed ID enables the analysis of the
consequences of the decision alternatives and performing effective
what-if analyses. The paper illustrates the construction and
analysis of IDs with two examples from the field of military
aviation.
How Simulation Languages Should Report Results: A Modest Proposal
Jamie R. Wieland (Illinois State University) and Barry L. Nelson (Northwestern University)
Abstract:
The focus of simulation software environments is on developing simulation models; much less consideration is placed on reporting results. However, the quality of the simulation model is irrelevant if the results are not interpreted correctly. The manner in which results are reported, along with a lack of standardized guidelines for reports, could contribute to the misinterpretation of results. We propose a hierarchical report structure where each reporting level provides additional detail about the simulated performance. Our approach utilizes two recent developments in output analysis: a procedure for omitting statistically meaningless digits in point estimates, and a graphical display called a MORE Plot, which conveys operational risk and statistical error in an intuitive manner on a single graph. Our motivation for developing this approach is to prevent or reduce misinterpretation of simulation results and to provide a foundation for standardized guidelines for reporting.
