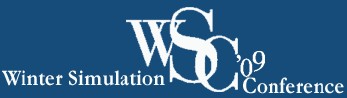 |
WSC 2009 Final Abstracts
|
Methodology - Risk Analysis Track
Tuesday 10:30:00 AM 12:00:00 PM
Efficient Monte Carlo Methods for Risk Analysis
Chair: Jeremy Staum (Northwestern University)
Estimating Expected Shortfall with Stochastic Kriging
Ming Liu and Jeremy Staum (Northwestern University)
Abstract:
We present an efficient two-level simulation procedure which uses stochastic kriging, a metamodeling technique, to estimate expected
shortfall, a portfolio risk measure. The outer level simulates financial scenarios and the inner level of simulation estimates
the portfolio value given a scenario. Spatial metamodeling enables inference about portfolio values in a scenario based on
inner-level simulation of nearby scenarios, reducing the required computational effort. Because expected shortfall involves the
scenarios that entail the largest losses, our procedure adaptively allocates more computational effort to inner-level simulation of those scenarios, which also improves computational efficiency.
Quantifying Supply Chain Disruption Risk Using Monte Carlo and Discrete-event Simulation
Amanda J. Schmitt and Mahender Singh (MIT Center for Transportation and Logistics)
Abstract:
We present a model constructed for a large consumer products company to assess their vulnerability to disruption risk and quantify its impact on customer service. Risk profiles for the locations and connections in the supply chain are developed using Monte Carlo simulation, and the flow of material and network interactions are modeled using discrete-event simulation. Capturing both the risk profiles and material flow with simulation allows for a clear view of the impact of disruptions on the system. We also model various strategies for coping with the risk in the system in order to maintain product availability to the customer. We discuss the dynamic nature of risk in the network and the importance of proactive planning to mitigate and recover from disruptions.
Estimating Mean of Non-linear Function of Conditional Expectation
Sandeep Juneja (Tata Institute) and Jeff Hong (Hong Kong University of Science and Technology)
Abstract:
Consider the problem of estimating the expectation of a non linear function of a conditional expectation. This function is allowed to be non-differentiable and discontinuous at a finite set of points to capture practical settings. We develop a nested simulation strategy to estimate this via simulation and identify bias and optimized mean square error allocation. We show that this mean square error converges to zero at the rate $\Gamma^{-2/3}$, as $\Gamma \rightarrow \infty$, where $\Gamma$ denotes the available computational budget. We also consider combining nested simulation technique with kernel based estimation methods. We note that while the kernel based method have a better convergence rate when the underlying random process has dimensionality less than or equals to three, pure nested simulation may be preferred when this dimension is above four.
Tuesday 1:30:00 PM 3:00:00 PM
Option Pricing and Sensitivity Estimation
Chair: Nan Chen (Chinese University of Hong Kong)
Multilevel Monte Carlo for Basket Options
Mike Giles (Mathematical Institute, Oxford University)
Abstract:
The multilevel Monte Carlo method has been previously introduced for the efficient pricing of options based on a single underlying quantity. In this paper we show that the method is easily extended to basket options based on a weighted average of several underlying quantities. Numerical results for Asian, look back, barrier and
digital basket options demonstrate that the computational cost to achieve a root-mean-square error of $\eps$ is $O(\eps^{-2})$.
This is achieved through a careful construction of the multilevel estimator which computes the difference in expected payoff when using different numbers of timesteps.
Sensitivity Analysis for Barrier Options
Yongqiang Wang, Michael C Fu, and Steven I Marcus (University of Maryland, College Park)
Abstract:
Barrier options are popular derivative securities with payoffs dependent
on whether or not an underlying asset crosses a barrier.
This paper presents a Monte Carlo simulation based method of sensitivity
analysis for barrier options based on smoothed perturbation analysis (SPA)
for a general form of discontinuous sample function payoffs.
The connection between the resulting SPA estimator
and the probability formula derived in Hong (2008) and its generalization in
Liu and Hong (2009) is explored.
Using a Brownian bridge result, the estimator is applied to continuously-monitored
barrier options with rebates. Illustrative simulation examples are provided.
A Wiener Measure Theoretic Approach to Pricing Extreme-Value-Related Derivatives
Nan Chen and Zhengyu Huang (Chinese University of Hong Kong)
Abstract:
Discretization schemes converge slowly when simulating extreme
values for stochastic differential equations. Using a Wiener measure
decomposition approach, this paper constructs an unbiased estimator
for pricing extreme-value-related derivatives, such as barrier and
lookback options, under a diffusion market model. A strong condition
on the coefficients is needed in the derivation of the estimator. We
also propose a truncation technique to remove this requirement and
show that the truncation error decays exponentially. The numerical
experiments reveal that this estimator is accurate and efficient.
Tuesday 3:30:00 PM 5:00:00 PM
Rare Event Simulation in Risk Analysis
Chair: Enlu Zhou (University of Illinois at Urbana-Champaign)
Rare Event Simulation for a Generalized Hawkes Process
Xiao-Wei Zhang, Peter Glynn, and Kay Giesecke (Stanford University) and Jose Blanchet (Columbia University)
Abstract:
In this paper we study rare event simulation for the tail
probability of an affine point process $(J_t)_{t\geq 0}$ that generalizes
the Hawkes process. By constructing a suitable exponential martingale,
we are able to construct an importance sampling algorithm that is
logarithmically efficient in the Gartner-Ellis asymptotic regime.
A Numerical Method for Financial Decision Problems Under Stochastic Volatility
Enlu Zhou (University of Illinois Urbana-Champaign) and Lin Kun, Michael C. Fu, and Steven I. Marcus (University of Maryland, College Park)
Abstract:
In many financial decision problems, such as portfolio optimization or hedging, the goal is to compute an optimal investment
strategy, in order to maximize expected utility or minimize expected risk. If the volatility of the risky asset(s) follows a
stochastic process and is not observable, the problems usually do not have analytical solutions. Hence, we propose an efficient
numerical method for these problems, based on a method developed recently for solving continuous-state partially observable
Markov decision processes. Numerical applications are presented and discussed for a problem of hedging European put and
call options.
